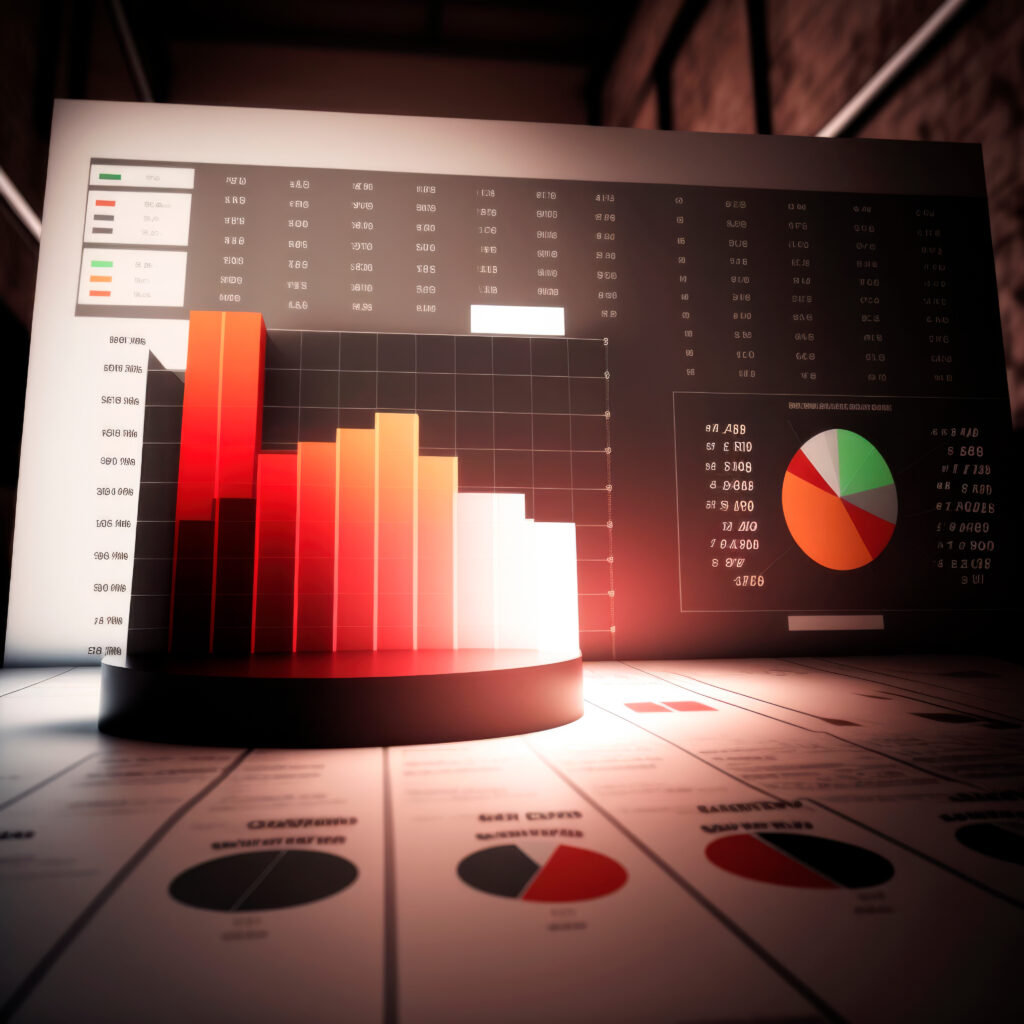
One fundamental statistical question for research areas such as precision medicine and health disparity is about discovering effect modification of treatment or exposure by observed covariates. This study from HIP Investigator Dr. Meggang Yu proposes a semiparametric framework for identifying such effect modification.
Instead of using the traditional outcome models, researchers directly posit semiparametric models on contrasts, or expected differences of the outcome under different treatment choices or exposures. Through semiparametric estimation theory, all valid estimating equations, including the efficient scores, are derived. Besides doubly robust loss functions, this approach also enables dimension reduction in presence of many covariates. The asymptotic and non-asymptotic properties of the proposed methods are explored via a unified statistical and algorithmic analysis. Comparison with existing methods in both simulation and real data analysis demonstrates the superiority of these estimators especially for an efficiency improved version.
Read More